AI in the Sky
Engineering professor Chaopeng Shen’s AI-based hydrological models helped PlantVillage teams in Africa combat a powerful locust plague and save the crops of thousands of farmers.
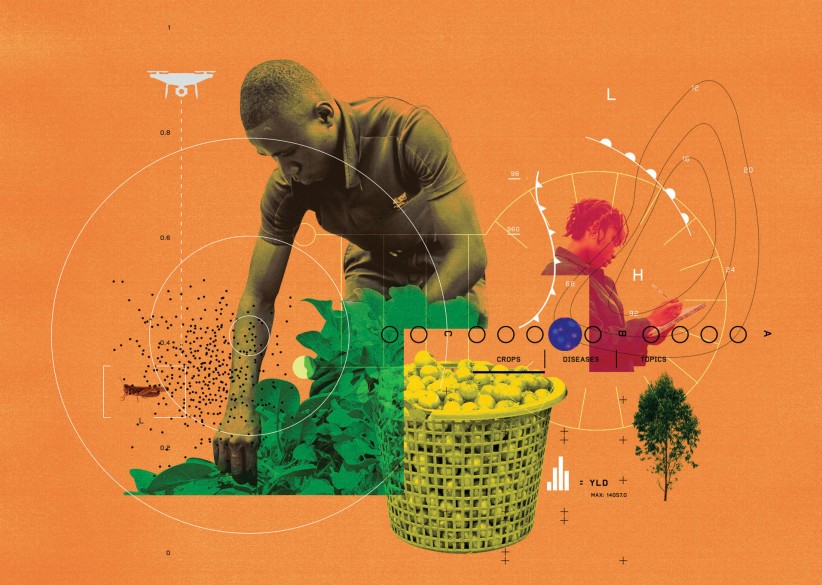
Engineering professor Chaopeng Shen’s AI-based hydrological models helped PlantVillage teams in Africa combat a powerful locust plague and save the crops of thousands of farmers.